A neural model of task compositionality with natural language instructions
- Datum: 20.06.2022
- Uhrzeit: 14:00 - 15:00
- Vortragende(r): Alexandre Pouget
- University of Geneva, Dept des Neurosciences Fondamentales, Switzerland
- Ort: Zoom
- Gastgeber: Peter Dayan (Philipp Schwartenbeck & Sebastian Bruijns)
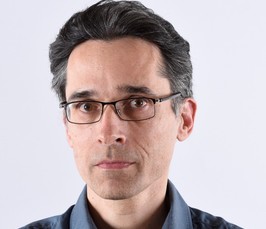
We present neural models of one of humans’ most astonishing cognitive
feats: the ability to interpret linguistic instructions in order to
perform novel tasks with just a few practice trials. We trained
recurrent neural networks on a set of commonly studied psychophysical
tasks, and receive linguistic instructions embedded by transformer
architectures pre-trained on natural language processing. Our best
performing models can perform an unknown task with a performance of 80%
correct on average based solely on linguistic instructions (i.e. 0-shot
learning). We found that the resulting neural representations capture
the semantic structure of interrelated tasks even for novel tasks,
allowing for the composition of practiced skills in unseen settings.
Finally, we also demonstrate how this model can generate a linguistic
description of a task it has identified using motor feedback, which,
when communicated to another network, leads to near perfect performance
(95%). To our knowledge, this is the first experimentally testable model
of how language can structure sensorimotor representations to allow for
task compositionality.